Analyses of metabolites and their ratios can be integrated with gene and protein expression data

Hatchery production of mussel larvae presents a number of biological and technological challenges that continue to hamper growth within the industry. Unexpected batch crashes are routinely observed in some hatcheries, and the causes are often not identified. When solutions are found and improvements made, these tend to be incremental and directed by technical managers.
Unfortunately, technical developments are poorly captured in the primary scientific literature and often not shared due to concerns over losing commercial advantage. In general, low production yields are hampered by high larval mortality rates that stem from the poor quality of broodstock, inadequate feeding regimes or unhealthy culture systems.
With the complex physical and biological factors within the larval-rearing process, it is not surprising larval cultivation still remains a hit-and-miss approach with variable chances of success. In this regard, metabolomics can provide a powerful means of identifying problems, and potentially could be used as an early warning system in subsequent cultures.
Metabolomics
Metabolomics is the study of chemical processes involving metabolites. As Wikipedia said, metabolomics is the “systematic study of the unique chemical fingerprints that specific cellular processes leave behind.” Metabolic profiling can give an instantaneous snapshot of the physiology of cells.
Because this is a new application in larval biology, there are only a few studies to illustrate its advantages for aquaculture. The authors recently performed a study using metabolomics to investigate intraspecific growth variations in New Zealand Greenshell™ Perna canaliculus mussel larvae.
Mussel aquaculture in New Zealand
In New Zealand, exports of P. canaliculus mussels represent the largest aquaculture sector by value and volume. Small-scale hatchery production of spat currently contributes only marginally toward the industry’s seed requirements. However, substantial research over the past decade has led to the ongoing development of selective breeding lines, establishment of a cryopreservation program, optimization of microalgae culture and larval-rearing procedures, and future strategies for extensive growth and upscaling of hatchery facilities.
The ability to provide consistency in larval quality and quantity is a critical step to achieve the commercial goal of successful large-scale production. Identification of biomarkers that reflect the immediate physiological condition of larvae has the potential to provide valuable tools that not only determine larval batch viability early on, but also identify the causes of poor production levels. This information can lead to better management decisions and improve overall hatchery operations.
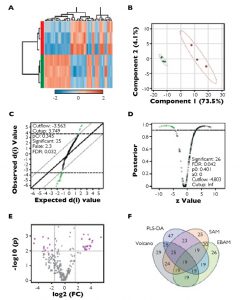
Study setup
In this study, the physiological condition of mussel larvae was used to assess larval quality during hatchery production. Mussel larvae were grown to umbo stage, separated based on relative sizes to divide poor-quality, slow-growing larvae from high-quality, fast-growing animals, and sampled over time. Six samples including about 80,000 pooled individuals from each of the two size fractions and three replicate rearing tanks were used for metabolite extractions via gas chromatography/mass spectrometry.
Metabolite peaks were identified, and their relative abundances were calculated. The dataset matrix was subjected to a variety of feature selection methods to identify potential biomarkers for discrimination of larval quality classes. Comprehensive Web-based analytical pipeline tools were used to statistically analyze the data sets.
To visualize feature differences between the larval quality classes, metabolite ratios were used to perform agglomerative hierarchical cluster analysis, displayed in dendrograms and heat maps. Four methods of feature reduction were used independently to minimize selection bias and provide robust criteria for assisting candidate biomarker identification.
Results
From the initial 253 metabolite ratios analyzed, candidate biomarkers were identified for assessing mussel larval quality using four independent feature selection methods: Volcano plot, partial least squares discriminant analysis (PLS-DA), significant analysis of microarrays (SAM) and empirical Bayesian analysis of microarrays (EBAM). These methods resulted in the selection of 19 common features.
Based on their performance, these were reduced to a final four metabolite ratios that were good candidates for assessing larval quality: alanine/succinate, glycine/succinate, myristic acid/succinate and pyroglutamate/succinate. Each of the identified ratios was substantially lower in the poor-quality larvae (Figure 1).
Comparing this group with the faster-growing cohort, the trend was characterized by elevated levels of succinate and simultaneous reductions in relative metabolite abundances of alanine, glycine, pyroglutamate and myristic acid. Based on the known functions of these metabolites, it was possible to identify potential biochemical pathways involved in larval performance. These pathways include energy metabolism, osmotic regulation, immune function and cell-to-cell communication.
Perspectives
While this study identified broad areas of larval physiology responsible for larval quality, the authors’ ongoing studies are providing more specific biomarkers intended to generate predictive models of larval performance. Furthermore, the aim is to eventually develop easy-to-use tool kits to evaluate the physiological state of larvae throughout the rearing process.
Further analysis of the single metabolites and their ratios will likely reveal additional information, and when integrated with gene and protein expression data, could provide new avenues for selective-breeding programs to consistently yield high-quality larvae. Supplementary experiments incorporating metabolomics-based approaches to investigate other measures of larval quality – such as health after immunological challenge and nutritional condition in response to diet manipulation – have the potential to offer a suite of biomarkers for a range of applications.
The use of metabolomics could also be applied to other areas of hatchery production to help close the loop on full life-cycle culture for many species. For example, routine production of high-quality gametes for successful fertilization and ongrowing could be achieved through better broodstock management and understanding of maternal provisioning, paternal effects and factors associated with high fecundity.
Authors
-
Andrea C. Alfaro, Ph.D.
Institute for Applied Ecology New Zealand
School of Applied Sciences
Auckland University of Technology
Auckland, New Zealand[122,110,46,99,97,46,116,117,97,64,111,114,97,102,108,97,97]
-
Tim Young, Ph.D.
Institute for Applied Ecology New Zealand
School of Applied Sciences
Auckland University of Technology
Tagged With
Related Posts

Innovation & Investment
Aquaculture in Canada: status, perspectives
Canada exports farmed seafood products to more than 22 countries and is the main seafood supplier to the U.S. market. Finfish, primarily salmon, production is strong and shellfish production is growing, but diversification will be imperative to maintain competitiveness.

Intelligence
Human enteric viruses in shellfish, part 3
Epidemiological studies have estimated that noroviruses are responsible for 60 percent to 80 percent of all foodborne outbreaks of gastroenteritis worldwide. Consumption of shellfish is one of three main transmission routes of norovirus infection.

Aquafeeds
The Bottom Line: Feeds and water quality revisited
Uneaten or undigested feed and their metabolic byproducts contribute to declines of water quality in aquaculture systems. Accordingly, feed and feeding techniques require continuous review. Reducing overfeeding is an important opportunity for improving feed conversion ratios.

Health & Welfare
Asepsis key to prevent contamination in shrimp hatcheries
Maintaining biosecurity and asepsis in larval shrimp production is a key component of the production chain in Ecuador, which requires the production of 5.5 billion larvae monthly from 300-plus hatcheries.