The different elements of the PFF production process

The main objectives of Precision Fish Farming (PFF) are to 1) improve accuracy, precision and repeatability in farming operations; 2) facilitate more autonomous and continuous biomass/animal monitoring; 3) provide more reliable decision support; and 4) reduce dependencies on manual labor and subjective assessments, and thus improve staff safety. Through these means, PFF will improve animal health and welfare while increasing the productivity, yield and environmental sustainability in commercial intensive aquaculture.
In defining PFF, it helps to envision fish farming as several cyclical operational processes realized in four phases where bio-responses in the cage are observed (observe phase) and interpreted (interpret phase), resulting in a foundation for making decisions (decide phase) on which actions to enforce (act phase) that in turn elicit a bio-response in the fish (Fig. 1). Similar cyclic concepts have been used to describe processes and products in other manufacturing industries.

Today, most tasks pertaining to the different phases are conducted manually (i.e. close to the center in Fig. 1). First, the farmer observes the fish via direct visual observation or with data acquisition tools such as cameras, the outcome of which is qualitative or quantitative information on the bio-responses of the fish. The farmer then uses primarily subjective experience to interpret this information, yielding a perception of the current state and condition of the fish.
These interpretations are then used as a foundation for making decisions concerning farming operations and management, which are then put into action by manually induced actions on the cage. Such decisions may be made based on the estimated present states or expected future states of the system, representing manual versions of the feedback and feed-forward principles in control engineering respectively.
Methods and tools for fish farming that apply technological solutions and/or automation principles to one, several or all the different phases of farming operations may be considered PFF approaches. The ultimate result of applying PFF to a particular operation will therefore be that the elements in that operation belonging to the different phases of fish farming operations are shifted from an experience-based to a knowledge-based regime (i.e. by moving from the center towards the outer edge in Fig. 1).
Status of PFF in present industry and research
Although the PFF concept has not previously been defined, many technological research efforts and equipment innovations for the fish aquaculture industry can be considered tools or components for developing PFF methods, and in a few cases are already PFF methods in their own right. In this article – adapted and summarized from the original publication – we provide an overview of the present status in this area, covering both industrial applications and research activities. Most relevant methods or concepts included here address a single phase in fish farming operations (Fig. 1), so each will be discussed separately.
Observe: Animal variables describing bio-responses
The general inability to use direct observation to make representative assessments of individual and population states underwater in fish farms means that fish farmers already depend on using technological solutions to monitor their animals. Submerged cameras are the most common tools found on fish farms today, and are used to observe the fish during production, with operators manually, and subjectively, analyzing behavior. Camera systems are useful platforms for automated fish monitoring by applying computer vision algorithms to the video stream.
The possibilities within computer vision techniques are expanding rapidly, both due to the development of enabling hardware such as camera and computer technology, and to the increased application of these technologies within the consumer electronics market. Computer vision methods can quantify several different animal variables in a fish farm setting, including clustering and movement, skin status, fish size, sea lice infestation levels and behavioral changes due to exposure to chemicals.
In addition, computer vision methods could monitor important properties of the physical environment, such as feed pellet quantities, and behavioral expressions observable above the water line such as surface activity. While the variation in technologies used to observe live fish in industry is limited, the methodological diversity within research is large, as researchers are constantly looking into new methods for collecting scientific data. In addition to cameras, active hydroacoustic devices are the most common technological tools used to study fish in aquaculture research.
The most frequent application of this technology has been the use of echo sounders to obtain echograms describing the vertical fish distribution and schooling density in the cage, which are animal variables that may be used to quantify the bio-response of fish to some treatment. More advanced hydroacoustic devices are already in use within other marine industry segments and could obtain additional animal variables from caged fish populations. For instance, splitbeam sonars can estimate swimming speeds and directions of individual fish within their sonar beam, while multibeam sonar systems can produce data on the 3D distribution and movements of the fish.
In addition, sonar-based systems may be used to assess individual fish sizes, given that it is possible to establish a relationship between the target strength of the fish and its mass or length. Passive hydrophones have also been used to provide information on Animal variables related to the behavior of several fish species including salmonids by recording the sounds emitted or generated by the fish. Considering that hydroacoustic devices (unlike cameras) are impervious to visibility conditions, this group of technologies could provide a useful foundation for PFF methods designed to acquire behavior-related animal variables for farmed fish populations.
Despite the considerable population sizes featured in modern fish farming, indicators of individual fish behavior may prove equally important in fish farming as population or group level animal variables. Acoustic fish telemetry is a method for remote sensing where individual fish are equipped with electronic transmitters containing sensors that measure some property in or near the fish, and that transmit raw or post-processed data wirelessly to submerged stationary receiver units using acoustic signals (i.e. sound waves). This technology is widely used for wild fish research but is also seeing increased usage within aquaculture-related research. Animal variables observed using this technology in a culture setting include individual depth movements, 3D positions, swimming activity levels, muscle activity levels and respiration rates or feed intake.
Farming operations at sea are subject to natural conditions at the site, as fish kept in sea-cages are exposed to conditions strongly influenced by the ambient environment (e.g. weather, water currents, sea states, temperatures, oxygen saturation, light levels and pollutants). Since many of these factors affect the growth, development and welfare of fish, data on the local ambient environment is important when selecting farming sites for salmon production. Furthermore, farmers increasingly want to monitor such conditions at their site also during production, as this information may be used as a foundation for making decisions concerning farm management, such as avoiding net manipulations when currents are strong, or reducing feeding when temperature decreases.

Table 1 summarizes some of the most common sensors and monitoring methods used to observe salmon in sea-cages today, including both industrially applied systems and solutions primarily used in research. Fig. 2 illustrates how a selection of these systems would be applied in a commercial cage.
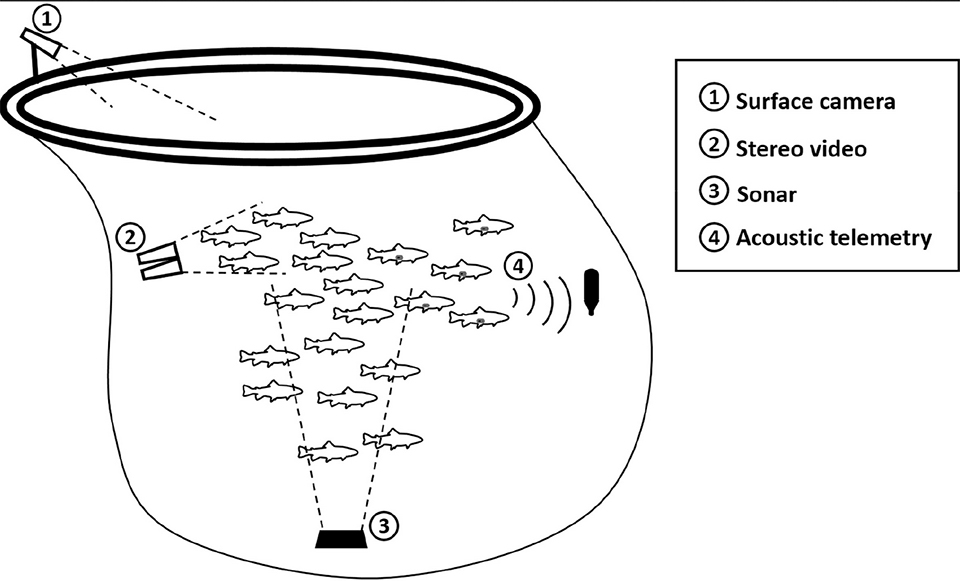
Interpret: Feature variables from animal variables
In the fish farming industry, the interpretation of animal observations is mainly conducted by individual farmers based on personal experience. Although ongoing innovations aspire to automate this process (e.g. systems for remote feeding operations that aggregate and present relevant data from different sources), the existing industrial foundation for automated interpretation of Feature variables is less established than it is for the acquisition of Animal variables. However, this also means that the unreleased potential for developing new PFF methods in this area is considerable. As production from the cage-based fish farming industry has increased, so has the extent of the research into obtaining a better understanding of the processes occurring in farmed populations. Aggregated knowledge on the different sub-mechanisms and bioprocesses occurring in commercial sea cages is therefore rapidly expanding.
However, before this knowledge can be put to use for decision support on a cage level, it needs to be structured to provide information relevant for the processes occurring in the cage. Mathematical modelling of systems dynamics is a tool commonly used for such inference, structuring and aggregating knowledge by synthesizing information from different subsystems into a complete system representation. A mathematical model of a dynamic system can often predict how the system will respond given a specific set of inputs and may estimate features of the system that are difficult or impossible to measure directly. In aquaculture research, mathematical models exist to estimate fish growth and behavior. Such models are good candidates as a foundation for PFF-methods aimed at interpretation, as they could predict or estimate properties of the fish based on measured inputs.
These inputs will often include various types of auxiliary data (e.g. environmental measurements, feed delivery and feeding schedules) required to drive the model dynamics but may also include measured animal variables that the model could then convert into feature variables more useful for decision support. For instance, a recent study sought to estimate the economic yield of a production operation by combining a model of sea bream growth with temperature as input with simulations of sales plans and strategies. Another example of such use of mathematical models could be to use a mathematical model to estimate feature variables such as the feeding activity of the fish, the distribution of waste material in the water, or vertical fish swimming speeds based on animal variables such as vertical distribution obtained with an echo sounder as input.
Mathematical models representing elements of the environment in production units also exist, covering subjects such as spatial and temporal feed distribution in sea-cages. If provided with sufficiently good input data, such models could estimate feature variables not directly associated with the fish but rather with the production environment.
The use of mathematical models to estimate unobserved states in complex systems has a long history within control engineering and is realized by including the mathematical model into an observer structure, either based on statistical methods or by using non-linear observer methods. Such applications allow the combination of existing knowledge (through mathematical models) with real-time data from sensors to provide better estimates than it is possible to obtain with either sensors or models alone.
Since caged fish production is a predominantly biological process, it is more difficult to measure the different states and processes directly than in more technically oriented industries. By this reasoning, it is likely that extensive use of estimators based on mathematical models will be necessary components when deriving the PFF methods of the future, as precision farming will require a better foundation for information than is available through present monitoring methods.
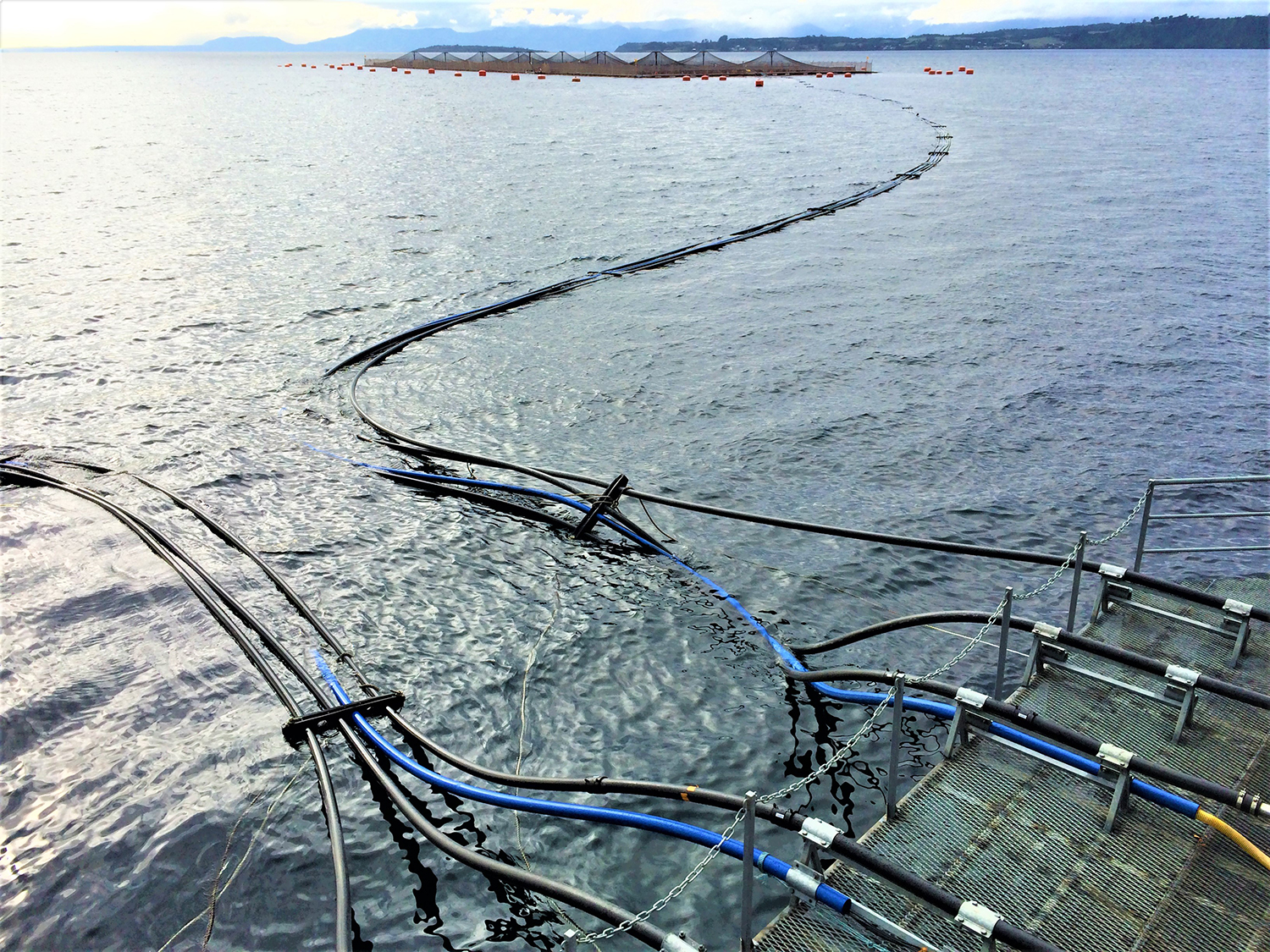
Decide: Target variables from feature variables
All important decisions in present day fish aquaculture are made by humans based on the interpretation of observations of the fish and other cage processes based on personal experience, and the use of protocols, legislation and recommendations on farm management. This will probably still be the case in the near future for fish farming operations, as making the “right” decision is a complex task that is difficult to assign to computer-based systems without running a risk of unforeseen and potentially undesirable side effects (e.g. suboptimal feeding due to limited data on fish responses). However, when fish farming operations are moved to more exposed and remote areas, limited human access will increase the need for autonomy in central tasks such as feeding.

Limited human presence also means that decision-making processes need to be at least partly automated. Although there exist no systems for automated decision making or decision support that are operative within the aquaculture industry, advances in artificial intelligence and information technology have led to the development of Decision Support Systems (DSS). A DSS is a computer tool that for a given situation or problem combines inputs (e.g. from sensors or mathematical models) and historical user experiences (i.e. from similar situations or problems previously experienced) into compound output values. DSS methods are used in several industries, including oil and gas, finance and medicine.
An example of research that aspires to go in the direction of DSS for fish farming is found in the proposed concept of using the fish as biological warning systems for monitoring seafood safety. Fish would be monitored online using technological methods (e.g. computer vision) to detect atypical behavior or responses in the fish that imply that the animals are affected by external perturbations, possibly indicating e.g. the presence of noxious substances.
Act: Manipulating system and eliciting desired bio-responses
Most actions that incite bio-responses at fish farms are manually controlled and often include the manual operation of mechanical equipment (e.g. winches, cranes, crowding nets and ropes). The task of converting a specific decision into the proper control signals or physical actions that elicit the desired response is assigned to a human operator. An important exception from this is the centralized feeding systems employed at most commercial fish farms. These systems are designed to convert decision level inputs such as cage specific feeding rates and feeding time schedules into the electrical signals (e.g. blower frequency, feed sluice opening rate and feed hose selector) required for the feeding process to achieve the desired system response.
In earlier days, most of the necessary underwater actions at fish farms were conducted by divers. Today it has become common to use remotely operated vehicles (ROVs) for such tasks, greatly reducing the risks of personnel injuries. Although ROVs are most often controlled by human pilots, recent research has demonstrated the possibility of using acoustic positioning methods and computer vision-based systems to improve the navigation of ROVs in and around cages, increasing the precision in remote operations. The use of this type of technology could also be extended to Autonomous Underwater Vehicles (AUVs) that move without human interference, and that could in turn be equipped to conduct minor repairs and other underwater tasks autonomously. AUVs have been used for different purposes within several industries, including hydrographic surveys, inspections and prospecting for oil and gas applications, ship hull inspections and military applications.

Closed-loop PFF applications
At present, there are no examples of systems that may be branded closed-loop PFF applications in farm-based fish aquaculture, encompassing the span from observing animal variables to actuation that elicits a bio-response in the fish. However, as the general level of technology in the world increases, the equipment commercially available to the fish farming industry also becomes more technically advanced and better able to handle more complex tasks. For instance, devices such as biomass frames may be argued to cover both the observe and interpret phases in the farming cycle (Fig. 1), as they optically scan the fish, estimate individual volumes based on the scanning data, and then estimate weight distributions based on these numbers. There are few examples of solutions that seek also to cover the decide and act phases, which often entail human intervention.
However, there are examples of such initiatives within research on live feed production, where different cybernetic methods (i.e. mathematical modelling, sensor technology and automated control) have been successfully applied to automatically control feeding and culture growth. Although this industrial segment differs greatly from cage-based fish farming in both scale and facilities, both are focused on the husbandry of live aquatic animals, and since such methods are possible to develop for live feed production, similar approaches may apply to farm-based fish production. This potential is even greater for land-based production of fish in onshore tanks, where the ability to control core aspects of the production environment (e.g. temperature, oxygen, flow) is substantially higher than for cage-based production.
Challenges for industrialization
Many of the technology principles that are potential tools in the fulfillment of industrial PFF applications have been used industrially and commercially in other market segments, and several have also seen some use within aquaculture. However, for many of these, there exist specific technical challenges related to the basic physics of the subsurface environment, properties pertaining to the selected sensing methods, or limitations of communication protocols when used in a fish farm setting. These challenges need to be overcome before a full step towards commercial exploitation in aquaculture is possible. Potential methods to handle such challenges may range from the implementation of new product features, through adjustment of system settings, to more strategic equipment placement (Table 2).
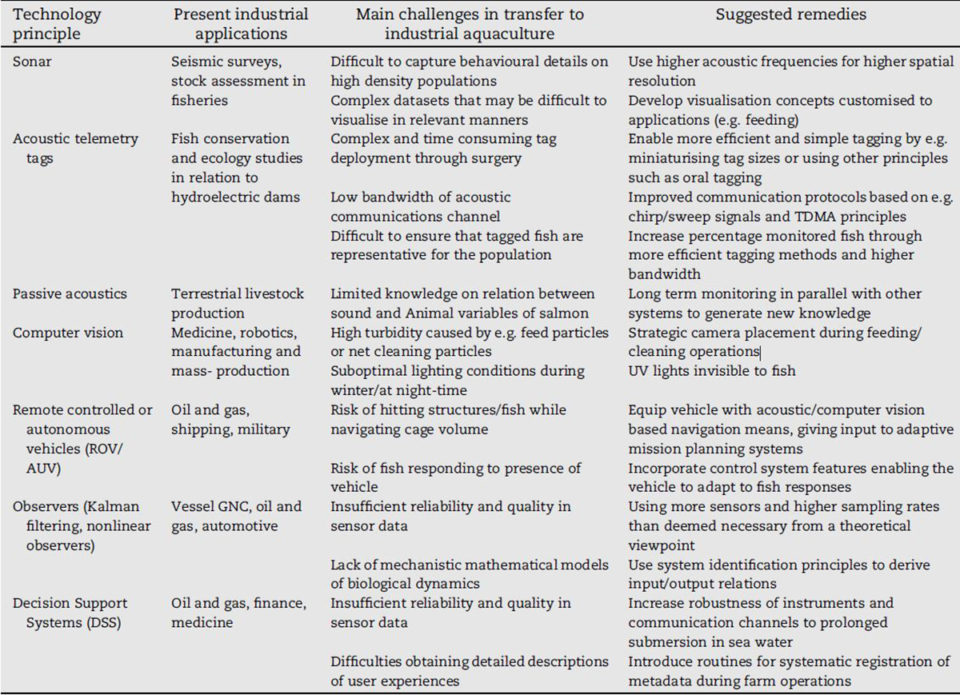
Acknowledgements
This study is the result of a strategic collaborative effort between the participating institutions and has not been funded through external grants. We dedicate this work to the late Professor Jens Glad Balchen (1926-2009) who first established the idea of applying cybernetic methods to the production and capture of aquatic organisms.
Editor’s note: This article has 14 co-authors (see tags for all author names) but only the affiliation and contact information for the first and corresponding author is listed.
References available from corresponding author.
Now that you've finished reading the article ...
… we hope you’ll consider supporting our mission to document the evolution of the global aquaculture industry and share our vast network of contributors’ expansive knowledge every week.
By becoming a Global Seafood Alliance member, you’re ensuring that all of the pre-competitive work we do through member benefits, resources and events can continue. Individual membership costs just $50 a year. GSA individual and corporate members receive complimentary access to a series of GOAL virtual events beginning in April. Join now.
Not a GSA member? Join us.
Author
-
Martin Føre, Ph.D.
Corresponding author
SINTEF Ocean
7465 Trondheim, Norway; and
NTNU Department of Engineering Cybernetics
7491 Trondheim, Norway[111,110,46,102,101,116,110,105,115,64,101,114,111,70,46,110,105,116,114,97,77]
Tagged With
Related Posts

Innovation & Investment
Algae innovators aim to freeze out early-stage shrimp losses
A greenhouse in Belgium believes its innovative shrimp feed product, made from freeze-dried microalgae, packs the necessary nutrients for the crustacean’s most vulnerable life stage: the first three days of its life.

Innovation & Investment
Eight digital technologies disrupting aquaculture
Eight digital technologies are disrupting aquaculture and having a profound impact on the way business operates – even displacing some established ones.

Innovation & Investment
Norway showcases halibut farm as sustainability star
Recognizing its potential as a sustainable fish producer, Norway highlights land-based halibut farmer Sogn Aqua in the debute of The Explorer digital showroom. The Advocate recently paid a visit to the farm in Ortnevik.

Responsibility
Calories count. Aquaculture makes them truly add up.
Salmon farms are quite different from terrestrial farms in providing both nutrition and calories. They produce stunningly more of both than their land-based counterparts.