Through GAIN project, funded by EU Horizon 2020 Fund, salmon farms use precision aquaculture to monitor shoaling and water conditions for improved farm management
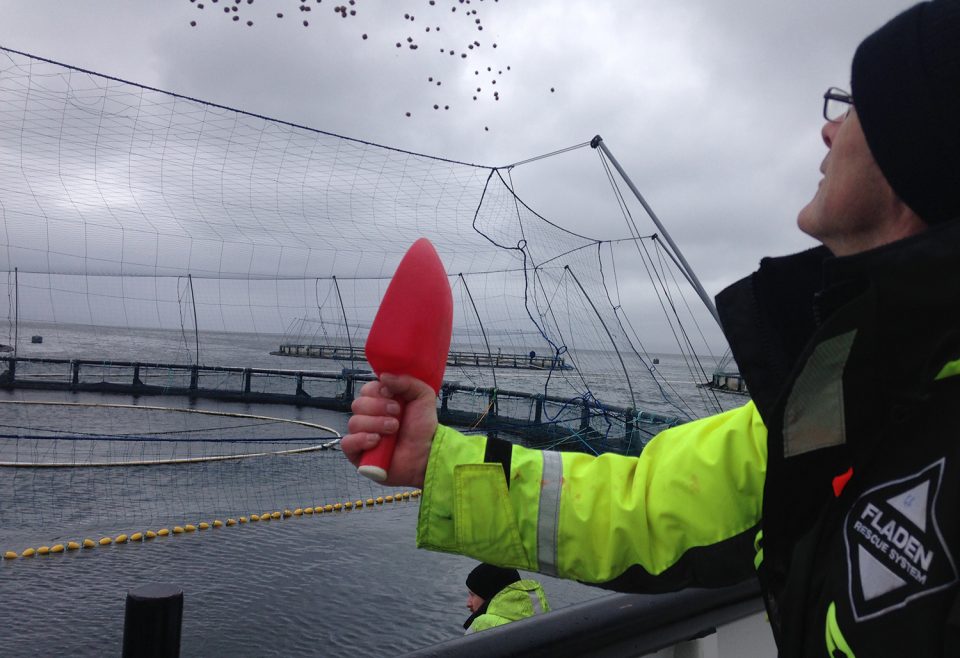
This is the third article in our series on precision aquaculture, part of a larger three-part series on sustainable aquaculture intensification in Europe. This work from the GAIN project is funded by the EU Horizon 2020 Fund. Each part in this series takes provides an introductory glance at three themes – precision aquaculture, sustainable feeds and the circular economy – and explains how outputs from the GAIN project are contributing to each, including the applicability to industry and governance. (Editor’s note: See part 1 on data and evidence-based management; part 2 on sea bass; part 3 on trout.)
Monitoring fish behavior in aquaculture systems is critical to ensuring optimal welfare and production. Behavioral changes in shoaling patterns or feeding habits can give farmers an early warning of potential health or water quality problems. Unlike with terrestrial animals, farmers must rely on underwater cameras to observe fish behavior below water. Even then, the analysis is often subjective, relying on farmer experience and familiarity with the animals to make an appropriate assessment. As farms scale up, this process becomes increasingly difficult. Our objective was to use machine learning to extract quantified and actionable insight from the diverse data collected by farmers.
To improve farmers’ understanding of fish behavior and its implications, the GAIN project collaborated to develop a precision aquaculture system using sensors and automatic machine learning. This system processes environmental and fish behavior patterns to extract insight on operational farm conditions and implications for fish welfare.
First, sensors were installed to collect information determining the vertical distribution of the fish in the cage, while a network of environmental sensors characterized local site conditions. “AutoAI” (automatic machine learning) collected and analyzed the data to develop high-quality models specific to each cage. Analysis and results were developed and disseminated using IBM Watson® Studio to provide a unified development environment for bespoke analysis specific to individual site characteristics.
To determine the distribution of fish in the cage, the sensors used hydroacoustic methods, where acoustic “backscattering” provided a proxy measure for density and distribution of marine animals. Hydroacoustic methods provide high-resolution data collected autonomously and automatically, regardless of water conditions or visibility. These non-invasive methods also reduce the need for handling fish and avoiding associated welfare issues.
We tested the machine learning framework at three salmon cage farms in Norway, Scotland and Canada. At each site, several environmental sensors were deployed to monitor a range of parameters, including temperature, dissolved oxygen and current speed. These were complemented with weather data from in-situ weather stations or from weather product data through IBM Environmental Intelligence Suite. This monitoring network provided a comprehensive overview of conditions within an extremely chaotic system consisting of up to 150,000 fish deployed in an exposed marine environment.
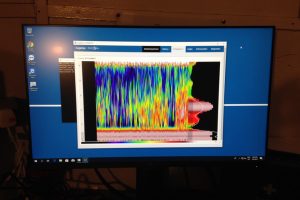
IBM AutoAI used statistical analysis and machine learning to examine the relationship between the vertical distribution of fish in a cage and environmental variables at the three geographically disparate sites and their evolution over time. We instructed the machine to consider temperature, dissolved oxygen, current speed, wind speed and salinity on an hourly basis, as literature suggests that these factors influence salmon behavior (and were available at the study sites).
The analysis presents an automated way to rapidly analyze ambient features influencing fish response and behavior in a cage. A better understanding of fish response can inform farmer’s actions to reduce fish stressors and improve welfare. Fig. 1 presents a density plot of daytime and nighttime fish positions for both the Canada and Norway sites. It illustrates detected differences in fish behavior between sites, where behavior at the Canada site varied between night and day, but not in the Norway sites. In this way, the system learns individual site conditions, giving each farmer more accurate predictions of welfare and production based on actual conditions.
Fish response based on thermoregulation (temperature variations), oxygen levels, or weather data can be used to inform feeding schedule, oxygen supplementation, or stocking densities to ensure fish health. This is particularly relevant to inform on deviations from ambient conditions and helping diagnose drivers of anomalous fish response. Taken together, information on environmental conditions and associated fish response can be used to generate a representation of fish welfare over the course of its cage life cycle. This can be integrated with fish traceability protocols (for example blockchain) to inform consumers on the conditions that farmed salmon were harvested to enhance sustainability and consumer trust.
This information can also be used to characterize site properties and how it compares to other locations. This could be particularly valuable when comparing different farm systems such as inshore and offshore and the associated operational implications. Considering environmental and operational aspects allows a greater understanding of multicriteria decisions that are inherent to site selection studies. For example, moving further offshore may reduce the risk of oxygen deficits due to increased wind and current speeds.
Read more about it in their recent publication.
Now that you've finished reading the article ...
… we hope you’ll consider supporting our mission to document the evolution of the global aquaculture industry and share our vast network of contributors’ expansive knowledge every week.
By becoming a Global Seafood Alliance member, you’re ensuring that all of the pre-competitive work we do through member benefits, resources and events can continue. Individual membership costs just $50 a year. GSA individual and corporate members receive complimentary access to a series of GOAL virtual events beginning in April. Join now.
Not a GSA member? Join us.
Authors
-
Feargal O’Donncha
Research Scientist
IBM Research -
Sonia Rey Planellas
Lecturer in Animal Behaviour and Welfare
Institute of Aquaculture
University of Stirling -
Alexandra Pounds
Assistant Researcher in Aquatic Food Security
Institute of Aquaculture
University of Stirling